Challenges and Limits of AI in Modern Orthopedic Surgery
- Data Quality Issues: AI relies on consistent, high-quality data, which is often limited.
- Interpretability: AI models are complex and can lack transparency.
- Cost Barriers: High-tech AI systems may increase costs for hospitals and patients.
- Patient Privacy Concerns: AI needs extensive patient data, raising privacy challenges.
- Ethical Questions: AI’s role in patient care introduces ethical considerations.
Artificial intelligence (AI) has revolutionized many aspects of modern healthcare, and orthopedic surgery is no exception. By assisting with diagnostics, surgical planning, and even intraoperative guidance, AI offers the potential for greater accuracy and personalization in patient care. However, while the benefits of AI in orthopedics are substantial, there are also considerable challenges and limitations that can impact its effectiveness and reliability. Understanding these challenges helps us approach AI with a balanced perspective, ensuring we leverage its strengths while remaining mindful of its current limitations.
Data Quality and Availability: The Foundation of Reliable AI
One of the most significant challenges in AI implementation for orthopedic surgery lies in data quality. AI models require vast amounts of high-quality data to function effectively, learning from complex datasets to identify patterns and generate accurate predictions. In orthopedics, this data includes medical imaging, patient demographics, surgical outcomes, and other health records.
Unfortunately, access to clean, consistent data is often limited. Variability in imaging standards, inconsistencies in electronic health records, and differences in data collection methods can lead to biased or incomplete datasets. This lack of standardized data can compromise AI’s accuracy, leading to erroneous or misleading predictions. In orthopedic surgery, where precision is paramount, even slight inaccuracies in data processing can have serious consequences for patient outcomes. Furthermore, data privacy regulations restrict access to medical data, adding another layer of complexity to AI model training and validation.
Diagnostic Challenges: Interpreting Complex Imaging Data
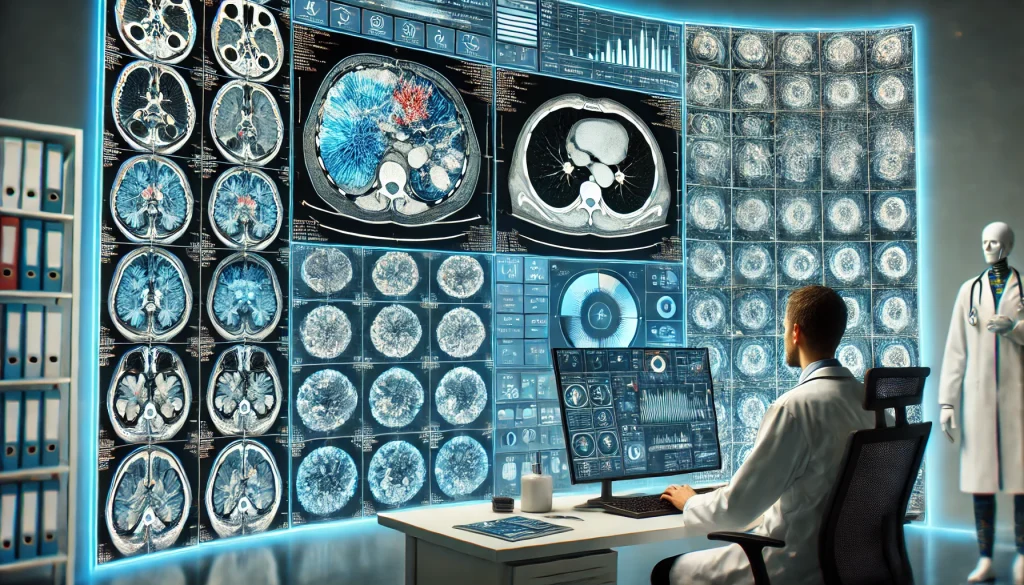
AI has demonstrated remarkable potential in interpreting medical imaging, assisting in diagnoses of fractures, joint degeneration, and even early-stage arthritis. However, orthopedic imaging presents unique challenges. Bones, joints, and surrounding tissues vary widely from patient to patient, and factors like age, activity level, and prior injuries can create subtle differences that are difficult for even advanced AI models to interpret accurately.
Orthopedic imaging also encompasses a variety of techniques—X-rays, MRIs, CT scans, and ultrasounds—each with unique imaging characteristics. AI models trained on one imaging type may struggle to interpret another accurately, limiting the versatility of these tools. Additionally, complex cases with multiple fractures or injuries involving both bone and soft tissue require nuanced interpretation, which AI is not always equipped to handle without extensive, condition-specific training. Consequently, reliance on AI for diagnostic support in orthopedics requires human oversight to ensure accurate assessment, particularly in complex or ambiguous cases.
Surgical Planning: Balancing AI Guidance with Surgeon Expertise
AI in surgical planning offers a promising way to personalize procedures, using patient data to recommend optimal implant selection, alignment, and other surgical variables. However, AI cannot yet account for all the factors that influence a successful outcome in orthopedic surgery. Every patient is unique, and factors like bone density, muscle structure, and surgical history can vary widely. These subtleties are not always fully captured in AI algorithms, making it essential for surgeons to interpret AI recommendations critically.
Another limitation is that AI-driven surgical plans are often based on population-level data, which may not account for individual patient variations. This “one-size-fits-all” approach can be problematic, as it might not suit a patient with an atypical anatomy or unique medical history. Surgeons, therefore, must weigh AI-driven insights against their own clinical judgment, making AI a supportive rather than a directive tool in surgical planning.
Intraoperative Guidance: Navigating Technical and Ethical Boundaries
Robotic-assisted surgery, powered by AI, provides orthopedic surgeons with unparalleled precision, especially in joint replacements and spinal fusions. However, intraoperative AI also comes with challenges. The operating room is a high-stakes, dynamic environment where unexpected complications can arise, requiring quick adjustments. While AI algorithms excel in stable conditions, they struggle to adapt to real-time changes, such as sudden bleeding or tissue variations that were not anticipated during preoperative planning.
AI-guided robotic systems also require highly skilled operators to manage them effectively, adding complexity to the surgical process. Moreover, reliance on AI for real-time decision-making raises ethical questions: if an AI system makes a miscalculation during surgery, determining accountability becomes a murky issue, particularly when patient safety is compromised.
Patient Privacy and Data Security: Protecting Sensitive Health Information
AI systems rely heavily on patient data for accurate functioning, especially in training models to make personalized predictions. However, this reliance brings significant privacy concerns. Medical records, imaging data, and even wearable device data are highly sensitive, and breaches of this information can have severe consequences for patients.
Healthcare providers must comply with stringent data protection laws, such as HIPAA in the United States and GDPR in the European Union, which place strict limits on how patient data can be stored, shared, and used. These regulations are essential for protecting privacy, but they also complicate AI development. The need for extensive de-identification and anonymization processes can reduce data quality, which impacts model accuracy. Data security also requires continuous monitoring, as cyberattacks targeting medical systems have increased, threatening patient privacy and trust in AI systems.
Cost and Accessibility: Making AI Feasible for All Patients
The cost of implementing AI-driven systems in orthopedic surgery is another barrier. High-tech AI applications require expensive equipment, such as robotic surgical assistants, advanced imaging tools, and secure data storage systems. For hospitals, especially those with limited budgets, investing in such technology can be prohibitively expensive, impacting their ability to offer AI-assisted orthopedic procedures to all patients.
Patients may also face higher costs associated with AI-based diagnostics and treatments, which can limit access for individuals without comprehensive insurance coverage. This cost barrier creates an inequality in healthcare, where only certain populations can benefit from advanced AI technologies. Bridging this gap requires both technological advancements to reduce costs and policy changes to make AI-driven care more accessible.
Limitations in Predictive Analytics: Navigating Uncertainty
Predictive analytics in orthopedics aims to forecast patient outcomes, such as recovery time or likelihood of complications. However, predictive models are not infallible. While these models use data to identify trends, they may overfit to specific datasets or underperform in patient populations that differ from the data used to train them.
For instance, predictive models trained primarily on data from a younger, healthier population may not perform as well for older patients with complex medical histories. Such limitations mean that predictive analytics should be used as an aid rather than an absolute determinant in clinical decision-making. Surgeons must remain cautious and interpret predictions within the context of each patient’s unique health profile.
Integrating AI into Clinical Workflows: Overcoming Resistance and Training Challenges
Integrating AI into orthopedic practices requires significant adjustments to existing clinical workflows. Healthcare professionals may resist adopting AI, particularly if they are unfamiliar with the technology or concerned about its impact on their roles. Training surgeons and staff to use AI effectively is a time-intensive process, requiring resources that some healthcare providers may lack.
There is also a learning curve associated with interpreting AI-generated data, especially for those unfamiliar with machine learning principles. The risk of misinterpretation can lead to poor decision-making, especially if healthcare professionals rely too heavily on AI insights without fully understanding them. Successful integration of AI into orthopedic workflows requires comprehensive training and a shift in clinical culture to embrace AI as a complementary tool.
Limited Interpretability of AI Models: The Black-Box Problem
Many AI systems, particularly those based on deep learning, operate as “black boxes,” meaning their internal decision-making processes are not easily understood, even by experts. In a field like orthopedics, where decisions directly impact patient health, this lack of transparency poses a challenge. Surgeons may hesitate to rely on AI tools if they do not fully understand how the system reaches its conclusions, especially when the stakes are high.
This black-box problem also complicates accountability, as it is difficult to trace the origins of an error if the AI’s processes are not transparent. To address this, researchers are working on developing interpretable AI models that allow healthcare professionals to understand the logic behind AI-driven recommendations, promoting confidence and reliability.
Ethical Considerations: Balancing Technology and Patient Care
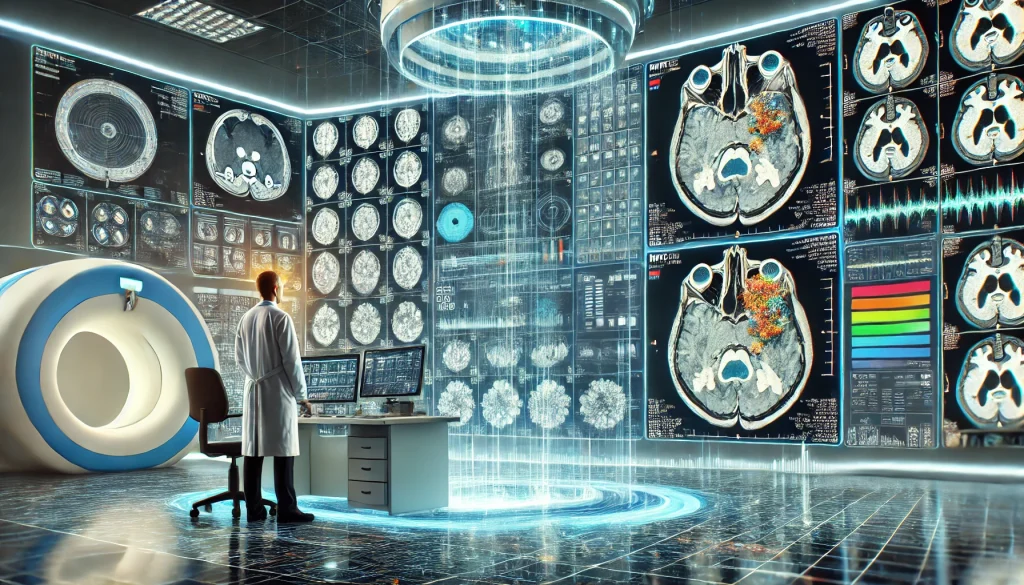
The rapid integration of AI in orthopedic surgery brings several ethical challenges, particularly regarding patient autonomy and consent. Patients may feel uncomfortable knowing that an AI system is involved in their diagnosis or surgical procedure, especially if they are not fully informed of its role. Additionally, ethical concerns arise around data use, as patients may not realize how extensively their data is used in AI training.
It is essential for healthcare providers to obtain informed consent and educate patients on how AI will be used in their care. Ethical AI use in orthopedics requires transparency, prioritizing patient well-being and autonomy throughout the decision-making process.
Future Prospects: Addressing Challenges to Unlock AI’s Full Potential
Despite the challenges, the future of AI in orthopedic surgery holds immense potential. Ongoing research aims to improve data quality, create more interpretable AI models, and reduce costs associated with AI-driven technology. By addressing the current limitations, we can pave the way for AI to transform orthopedic surgery in ways that prioritize patient safety, accessibility, and precision.
As AI continues to advance, it promises to offer increasingly sophisticated support to orthopedic surgeons. However, this progress must be approached carefully, with a focus on overcoming these challenges to ensure that AI can fulfill its role in providing safe, effective, and equitable healthcare.
Top 10 Real-Life Use Cases: Challenges and Limitations of AI in Orthopedic Surgery
AI has become a valuable tool in orthopedic surgery, offering potential benefits in diagnostics, surgical planning, and post-operative care. However, each advancement also brings its own set of challenges and limitations. In this article, we explore ten real-life use cases in which AI is applied in orthopedic surgery and examine the specific challenges, limitations, and benefits associated with each scenario.
1. Data-Driven Diagnosis of Bone Fractures
Challenges with Data Quality and Consistency
AI in diagnosing bone fractures uses imaging data to identify fractures and even subtle cracks that may be missed by human eyes. However, variations in image quality, angles, and patient positioning create challenges. AI requires consistent, high-quality data to deliver accurate results, which is not always available.
Benefits of AI in Diagnosis
- Improved Detection: AI can detect fractures that might be missed in initial exams, reducing diagnostic errors.
- Faster Diagnosis: AI speeds up the diagnostic process, leading to quicker treatment decisions.
2. Early Detection of Osteoarthritis
Limitations in Interpreting Subtle Degenerative Changes
Detecting osteoarthritis early is crucial for effective intervention. AI can identify early-stage degenerative changes in cartilage that may not be visible to the naked eye. However, these subtle changes can vary widely among individuals, making it challenging for AI to distinguish between normal aging and pathological changes accurately.
Benefits of Early Detection
- Timely Intervention: Early detection allows for preventive measures, potentially delaying the progression of osteoarthritis.
- Enhanced Patient Outcomes: Patients receive timely treatments, such as lifestyle changes or targeted therapies, which can improve quality of life.
3. Personalized Surgical Planning for Joint Replacements
Challenges in Addressing Unique Patient Variations
AI assists in joint replacement surgeries by recommending implant sizes and alignment based on individual anatomy. However, every patient’s body is unique, with specific muscle and bone variations that may not fit AI’s general recommendations. This lack of personalized data can result in inaccuracies.
Benefits of AI-Driven Surgical Planning
- Optimized Implant Fit: AI can recommend the best options based on standard anatomical data, potentially improving implant longevity.
- Reduced Risk of Misalignment: AI helps reduce common issues, such as misalignment, by assisting in planning accurate cuts and placements.
4. AI-Guided Spinal Surgery
Limitations in Managing Complex Anatomy and Risk Factors
Spinal surgery involves a high level of complexity, with delicate neural structures and potential for serious complications. AI models face challenges in adapting to spinal anomalies or cases with severe deformities, as these cases require highly individualized planning that AI cannot fully accommodate.
Benefits of AI in Spinal Surgery
- Enhanced Precision: AI helps surgeons plan with greater precision, reducing the risk of complications in straightforward cases.
- Improved Safety: AI-guided procedures are less invasive, potentially leading to shorter recovery times and reduced post-operative pain.
5. Predictive Analytics for Complication Risks
Challenges in Data Privacy and Security
Predictive analytics can assess risk factors for post-surgical complications, such as infection or delayed healing. However, using large datasets of patient information poses privacy risks. Regulations like GDPR in Europe and HIPAA in the United States add layers of complexity to how patient data can be used, stored, and shared.
Benefits of Predictive Analytics
- Targeted Preventive Measures: Surgeons can implement specific strategies to minimize the likelihood of complications.
- Better Patient Outcomes: By addressing potential risks proactively, healthcare teams can enhance recovery experiences and outcomes.
6. AI-Driven Intraoperative Guidance for Robotic-Assisted Surgery
Real-Time Adaptation Challenges in the Operating Room
Robotic systems equipped with AI provide valuable support during surgery, helping with precise cuts and alignment. However, unexpected events, such as bleeding or patient movement, pose challenges for AI, which may not be able to adapt quickly to these changes.
Benefits of Robotic Assistance
- Increased Accuracy: Robots assist surgeons in making precise incisions and placements, especially in joint replacements.
- Minimized Trauma: With greater control and accuracy, these procedures are often less invasive, reducing recovery time.
7. Customized Rehabilitation Plans Based on Predictive Models
Limitations in Predicting Recovery for Diverse Patient Profiles
AI-driven rehabilitation plans use predictive models to create tailored exercises for patients post-surgery. However, these models often lack data on diverse patient populations, such as those with pre-existing conditions or elderly patients, making it difficult to generalize recovery timelines.
Benefits of AI-Driven Rehabilitation
- Faster Recovery: Customized rehabilitation plans improve patient engagement and adherence, leading to quicker recovery.
- Injury Prevention: Personalized programs minimize the risk of overexertion or re-injury during rehabilitation.
8. Pain Management Recommendations Using AI Algorithms
Challenges in Accounting for Individual Pain Thresholds
AI can assist in creating pain management plans by predicting peak pain periods and suggesting optimal medication schedules. However, pain is highly subjective, and individual thresholds vary significantly. AI struggles to account for these personal differences, which can lead to over- or under-treatment.
Benefits of AI in Pain Management
- Reduced Dependency on Opioids: AI helps manage pain effectively with balanced doses, reducing reliance on opioid medications.
- Enhanced Patient Comfort: Patients experience a smoother recovery with more personalized pain relief options.
9. Optimizing Operating Room Scheduling with Predictive Analytics
Limitations in Handling Multiple Variables and Unexpected Delays
AI helps optimize operating room schedules by estimating surgery durations and resource needs. However, unexpected complications or delays make it challenging for AI to adjust schedules in real-time. Complex cases that require extra time or resources can disrupt the entire schedule.
Benefits of Predictive OR Scheduling
- Reduced Patient Waiting Times: Efficient scheduling improves patient satisfaction by minimizing delays.
- Increased Access to Surgery: Optimized scheduling can increase the number of procedures completed each day, helping patients receive care faster.
10. Patient Education and Engagement Through AI-Powered Tools
Challenges in Building Trust and Understanding with Patients
AI-powered tools provide patients with tailored educational resources, guiding them through the surgical process, rehabilitation, and follow-up care. However, some patients may feel uneasy relying on AI for medical guidance, leading to trust issues and reluctance to follow AI-generated recommendations.
Benefits of AI in Patient Education
- Better Informed Patients: AI tools provide patients with clear, accessible information, helping them make educated decisions about their care.
- Improved Engagement: Patients who understand their treatment are more likely to adhere to pre- and post-operative plans, supporting successful outcomes.
FAQ on Challenges and Limitations of AI in Orthopedic Surgery
How is AI used in orthopedic surgery today?
AI is used in various stages of orthopedic surgery, including diagnostics, surgical planning, and intraoperative guidance. It analyzes imaging data, recommends surgical approaches, and assists in creating personalized rehabilitation plans.
What are the main challenges of using AI in orthopedic surgery?
One major challenge is data quality. AI systems rely on high-quality, consistent data, which can vary across patients and hospitals. Other challenges include high costs, privacy concerns, and the need for specialized training to interpret AI results accurately.
Can AI replace surgeons in orthopedic procedures?
AI is designed to support surgeons, not replace them. It provides valuable insights, such as risk assessments and surgical guidance, but surgeons are essential for making complex, real-time decisions that require expertise and adaptability.
How does AI improve diagnostic accuracy in orthopedics?
AI can detect subtle patterns in imaging scans, such as small fractures or early signs of osteoarthritis, that may be hard to spot with the naked eye. However, its accuracy depends heavily on the quality and consistency of the data used to train it.
What are the limitations of AI in surgical planning?
AI-driven surgical plans are often based on population-level data, which may not account for individual patient differences. This can limit its ability to fully personalize surgical recommendations, making the surgeon’s expertise vital for final decisions.
Is AI effective in predicting recovery times?
AI models can analyze patient data to predict general recovery timelines, but recovery varies widely among individuals. Factors like age, pre-existing conditions, and overall health can affect predictions, so these estimates should be used as guides rather than absolute expectations.
How does AI help with pain management after surgery?
AI systems can track patient pain levels over time, helping doctors anticipate peak pain periods and adjust medication schedules. This approach supports balanced pain relief, though individual pain thresholds can vary, requiring personalized adjustments.
What privacy issues arise with AI in orthopedic surgery?
AI systems rely on patient data to make accurate predictions, raising concerns about data privacy and security. Hospitals must comply with privacy laws like HIPAA and GDPR, which require secure storage and limited sharing of patient data.
Why is data quality so important for AI in orthopedics?
Data quality affects how accurately AI models perform. Poor or inconsistent data can lead to biased or unreliable predictions, which is risky in orthopedic surgery, where precise information is essential for treatment planning and patient care.
Are there cost barriers to implementing AI in orthopedic surgery?
Yes, AI technology requires significant investment in software, equipment, and specialized training. This can make it challenging for hospitals with limited budgets to adopt AI, potentially limiting patient access to AI-assisted care.
Does AI impact the patient experience in orthopedic surgery?
AI has the potential to improve certain aspects of the patient experience, such as faster diagnostics and more tailored care plans. However, some patients may feel uneasy about the technology and need additional education to understand its role in their treatment.
What role does AI play in robotic-assisted surgeries?
In robotic-assisted surgeries, AI supports the robot by providing data-driven guidance on incisions, alignment, and positioning. This allows for precise movements that can reduce tissue damage and support quicker healing.
Is there a learning curve for surgeons using AI in orthopedics?
Yes, integrating AI into practice requires training to interpret AI data accurately and manage AI-driven tools effectively. This learning curve varies depending on the surgeon’s familiarity with AI technology and their experience in orthopedic procedures.
Can AI predict surgical complications accurately?
AI models can assess risk factors for complications like infection or delayed healing, providing surgeons with helpful insights. However, they are not foolproof and should be used as part of a comprehensive risk assessment that includes the surgeon’s clinical expertise.
What is the future of AI in orthopedic surgery?
As AI technology advances, it may become even more precise and accessible, supporting better patient outcomes and potentially lowering healthcare costs. However, challenges like data quality, privacy, and ethical concerns will need continued attention as the technology develops.