Overcoming Obstacles in Surgical Deep Learning
- Ensuring high-quality and diverse data collection
- Addressing algorithmic bias and fairness
- Integrating seamlessly with existing systems
- Managing real-time data processing requirements
- Navigating ethical and legal considerations
Introduction
Deep learning is transforming the field of surgery, offering unprecedented precision and real-time insights. However, implementing deep learning in surgical practices comes with significant challenges. This article explores the various obstacles and considerations that must be addressed to fully realize the potential of deep learning in surgery.
Data Quality and Quantity
Ensuring High-Quality Data
For deep learning models to be effective, they require large amounts of high-quality data. In surgery, collecting such data can be challenging due to patient privacy concerns and the variability in surgical procedures.
Key Points:
- Data Collection: Gathering extensive and diverse surgical data.
- Data Quality: Ensuring accuracy and consistency in data.
- Privacy Concerns: Protecting patient confidentiality.
Algorithm Bias
Addressing Inequities in Data
Deep learning models can inadvertently perpetuate biases present in the training data. This can lead to unequal treatment outcomes, particularly for underrepresented patient groups.
Key Points:
- Bias Identification: Detecting biases in datasets.
- Diverse Data: Using representative datasets.
- Regular Audits: Continuously monitoring and adjusting algorithms.
Integration with Existing Systems
Seamless Technology Integration
Integrating deep learning technologies with existing surgical systems and workflows can be complex. Compatibility issues and the need for significant infrastructure upgrades can pose substantial hurdles.
Key Points:
- Compatibility: Ensuring new technologies work with existing systems.
- Infrastructure: Upgrading necessary hardware and software.
- Workflow Disruption: Minimizing disruptions to surgical practices.
Real-Time Processing
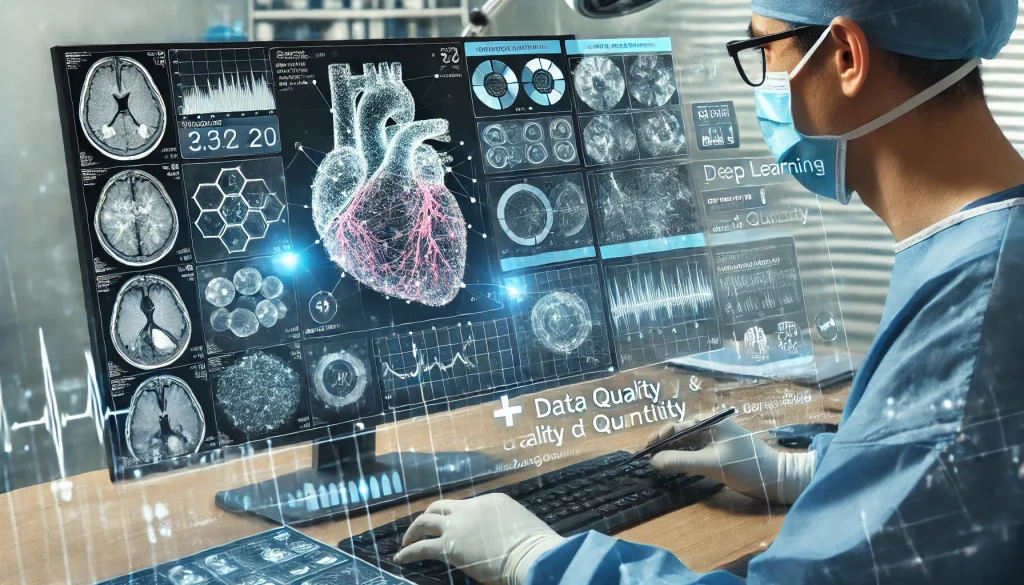
Ensuring Speed and Accuracy
Deep learning models must process data in real-time to provide valuable insights during surgery. Achieving this requires high computational power and efficient algorithms.
Key Points:
- Computational Power: Ensuring adequate processing capabilities.
- Algorithm Efficiency: Developing fast and accurate models.
- Latency Reduction: Minimizing delays in data processing.
Ethical and Legal Considerations
Navigating Ethical Challenges
The use of deep learning in surgery raises several ethical and legal issues, including patient consent, data privacy, and accountability for decisions made by AI.
Key Points:
- Informed Consent: Ensuring patients understand and agree to data use.
- Data Privacy: Protecting patient information.
- Accountability: Clarifying responsibility for AI-assisted decisions.
Training and Skill Development
Preparing Surgeons for New Technologies
Surgeons and healthcare professionals must be trained to effectively use deep learning tools. This requires comprehensive education and ongoing skill development.
Key Points:
- Training Programs: Implementing thorough training initiatives.
- Continuous Education: Providing ongoing learning opportunities.
- Skill Assessment: Regularly evaluating proficiency with new tools.
Cost and Accessibility
Managing Financial and Logistical Barriers
Implementing deep learning in surgery can be expensive, limiting its accessibility. Ensuring equitable access to these technologies is a significant challenge.
Key Points:
- Cost Management: Controlling the expenses of new technologies.
- Funding: Securing financial support for implementation.
- Accessibility: Ensuring broad access to advanced tools.
Reliability and Robustness
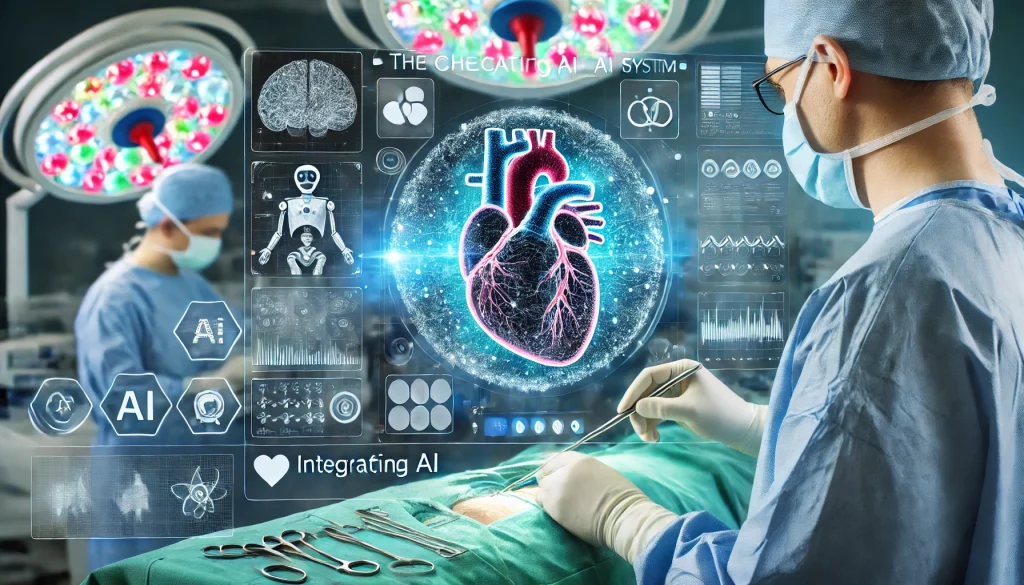
Ensuring Consistent Performance
Deep learning models must be reliable and robust, performing consistently across various surgical scenarios. Achieving this requires extensive testing and validation.
Key Points:
- Model Validation: Conducting thorough testing of models.
- Scenario Testing: Ensuring performance across diverse conditions.
- Reliability: Maintaining consistent and dependable outcomes.
Patient Trust and Acceptance
Building Confidence in AI
Gaining patient trust and acceptance of deep learning technologies in surgery is crucial. Clear communication and demonstrated success are key to building this trust.
Key Points:
- Communication: Explaining the benefits and limitations of AI.
- Transparency: Being open about how technologies are used.
- Success Stories: Sharing successful case studies.
Continuous Improvement
Evolving with Technological Advances
Deep learning technologies must continuously evolve to keep pace with advancements in medical science and technology. This requires a commitment to ongoing research and development.
Key Points:
- Research: Investing in continuous innovation.
- Development: Improving models and algorithms.
- Adaptability: Keeping up with technological changes.
Conclusion
While deep learning holds immense potential for transforming surgery, addressing these challenges is crucial for its successful implementation. By focusing on data quality, algorithm bias, system integration, real-time processing, ethical considerations, training, cost, reliability, patient trust, and continuous improvement, we can harness the power of deep learning to revolutionize surgical practices and improve patient outcomes.
Top 10 Real-Life Use Cases: Challenges in Deep Learning for Surgery
Ensuring High-Quality and Diverse Data Collection
Real-Life Scenario: Multi-Center Data Collaboration
Hospitals collaborate across multiple centers to gather extensive and diverse surgical data. This helps create robust datasets necessary for training accurate deep learning models.
Benefits:
- Data Diversity: Ensures models are trained on varied patient demographics.
- Accuracy: Improves the accuracy of predictive models.
- Bias Reduction: Reduces the risk of algorithmic bias.
Addressing Algorithmic Bias and Fairness
Real-Life Scenario: Bias Audits and Adjustments
A healthcare institution regularly audits their deep learning models for bias, using diverse datasets to identify and correct any disparities in treatment outcomes.
Benefits:
- Fair Treatment: Ensures equitable healthcare for all patient groups.
- Trust Building: Increases patient trust in AI-assisted surgery.
- Outcome Improvement: Enhances overall treatment effectiveness.
Integrating Seamlessly with Existing Systems
Real-Life Scenario: Step-by-Step Integration
A hospital integrates deep learning technologies gradually, ensuring compatibility with existing surgical systems and workflows. They conduct pilot programs before full-scale implementation.
Benefits:
- Minimal Disruption: Reduces workflow interruptions.
- Compatibility: Ensures new technologies work seamlessly with current systems.
- Smooth Transition: Facilitates a smoother transition to advanced technologies.
Managing Real-Time Data Processing Requirements
Real-Life Scenario: High-Performance Computing Deployment
A surgical center invests in high-performance computing infrastructure to support real-time data processing, enabling deep learning models to provide immediate insights during surgery.
Benefits:
- Real-Time Insights: Offers immediate feedback during procedures.
- Improved Precision: Enhances the accuracy of surgical actions.
- Timely Decision-Making: Supports quick and informed decisions.
Navigating Ethical and Legal Considerations
Real-Life Scenario: Establishing Ethical Guidelines
A healthcare network establishes an ethics committee to oversee the use of deep learning in surgery, ensuring compliance with legal standards and ethical practices.
Benefits:
- Ethical Compliance: Adheres to ethical and legal standards.
- Patient Trust: Builds trust in the use of new technologies.
- Clear Accountability: Defines responsibilities and maintains transparency.
Ensuring Adequate Training and Skill Development
Real-Life Scenario: Comprehensive Training Programs
A medical school implements a comprehensive training program for surgeons, focusing on the effective and ethical use of deep learning tools in surgery.
Benefits:
- Proficiency: Enhances surgeons’ skills with new technologies.
- Ongoing Education: Keeps healthcare professionals updated.
- Patient Safety: Ensures safe and effective use of deep learning tools.
Managing Costs and Accessibility
Real-Life Scenario: Securing Funding and Partnerships
A hospital secures funding from government grants and private partnerships to implement deep learning technologies, ensuring that these advancements are accessible to all patients.
Benefits:
- Financial Support: Provides necessary funding for implementation.
- Broad Accessibility: Ensures equitable access to advanced tools.
- Sustainable Implementation: Supports long-term use of new technologies.
Ensuring Reliability and Robustness
Real-Life Scenario: Extensive Testing and Validation
A research team conducts extensive testing and validation of deep learning models across various surgical scenarios to ensure consistent and reliable performance.
Benefits:
- Consistency: Ensures reliable outcomes in diverse conditions.
- Dependability: Builds confidence in the use of deep learning tools.
- Performance Assurance: Guarantees robust model performance.
Building Patient Trust and Acceptance
Real-Life Scenario: Transparent Communication
A hospital communicates openly with patients about the benefits and limitations of deep learning technologies, providing clear and accessible information to build trust.
Benefits:
- Patient Confidence: Increases acceptance and trust in AI-assisted surgery.
- Informed Decisions: Helps patients make informed choices.
- Transparency: Maintains open communication about technology use.
Continuous Improvement and Adaptation
Real-Life Scenario: Ongoing Research and Development
A healthcare institution commits to continuous research and development, regularly updating their deep learning models to incorporate the latest medical advancements and technologies.
Benefits:
- Innovation: Keeps surgical practices at the cutting edge.
- Improvement: Continuously refines and enhances model accuracy.
- Adaptability: Ensures the technology evolves with medical advancements.
FAQ about Challenges in Deep Learning for Surgery
What is deep learning in surgery?
Deep learning in surgery uses advanced algorithms to analyze large amounts of data, providing real-time insights and decision-making support during surgical procedures.
Why is data quality important in deep learning for surgery?
High-quality data ensures that deep learning models are accurate and reliable. Poor data quality can lead to incorrect predictions and outcomes.
How can algorithmic bias affect surgical outcomes?
Algorithmic bias can lead to unequal treatment outcomes for different patient groups, potentially causing harm to underrepresented populations.
What challenges exist in integrating deep learning with existing surgical systems?
Integration challenges include compatibility with current systems, the need for infrastructure upgrades, and minimizing disruptions to surgical workflows.
Why is real-time data processing crucial in surgical applications?
Real-time data processing provides immediate insights and feedback during surgery, allowing for precise and timely decision-making.
How are patient privacy and data security maintained in deep learning applications?
Patient privacy is maintained through advanced encryption methods and strict access controls, ensuring that sensitive information is protected.
What ethical considerations are associated with deep learning in surgery?
Ethical considerations include informed consent, data privacy, and accountability for decisions made by AI systems.
How is the bias in deep learning algorithms addressed?
Bias is addressed by using diverse datasets, conducting regular audits, and making necessary adjustments to the algorithms.
What training is required for surgeons to use deep learning tools effectively?
Surgeons need comprehensive training on the capabilities and limitations of deep learning tools, along with ongoing education to stay updated.
How can the cost of implementing deep learning in surgery be managed?
Costs can be managed by securing funding from grants and partnerships, and by ensuring that the benefits of the technology justify the investment.
What measures are taken to ensure the reliability of deep learning models in surgery?
Reliability is ensured through extensive testing and validation across various surgical scenarios, guaranteeing consistent performance.
How do healthcare providers build patient trust in deep learning technologies?
Trust is built through transparent communication about the benefits and limitations of the technology, and by sharing successful case studies.
What legal standards must be followed when using deep learning in surgery?
Legal standards include data protection regulations such as HIPAA, which govern the use and protection of patient data.
How do deep learning models adapt to new medical advancements?
Deep learning models adapt through continuous research and development, incorporating the latest medical advancements and technologies.
What are the main benefits of using deep learning in surgery?
The main benefits include improved precision, real-time decision-making support, and the ability to analyze large datasets for better outcomes.