Navigating Predictive Analytics Challenges in Surgery
- Increasing need for standardized data collection.
- Addressing algorithmic bias and fairness.
- Balancing real-time processing with accuracy.
- Enhancing patient privacy and data security.
- Navigating complex legal and regulatory landscapes.
- Integrating predictive tools into clinical workflows effectively.
Introduction
Predictive analytics holds transformative potential in the field of surgery, offering insights that can improve patient outcomes, optimize surgical planning, and reduce complications. However, the integration of predictive analytics into surgical practice is not without its challenges. These obstacles must be addressed to fully harness the power of predictive models in a way that is safe, ethical, and effective.
Data Quality and Availability
Inconsistent Data Collection
The effectiveness of predictive analytics relies heavily on the quality of the data fed into the models. Inconsistent data collection methods across different healthcare providers can lead to gaps or inaccuracies in the data, undermining the reliability of predictions. Standardizing data collection processes is crucial to ensure that the information used is both comprehensive and accurate.
Limited Access to Comprehensive Data
Not all healthcare facilities have access to large datasets necessary for building robust predictive models. Smaller institutions or those in under-resourced areas may lack the data needed to make accurate predictions. Collaboration between institutions could help in sharing data and resources, ensuring more inclusive access to predictive analytics tools.
Algorithmic Bias
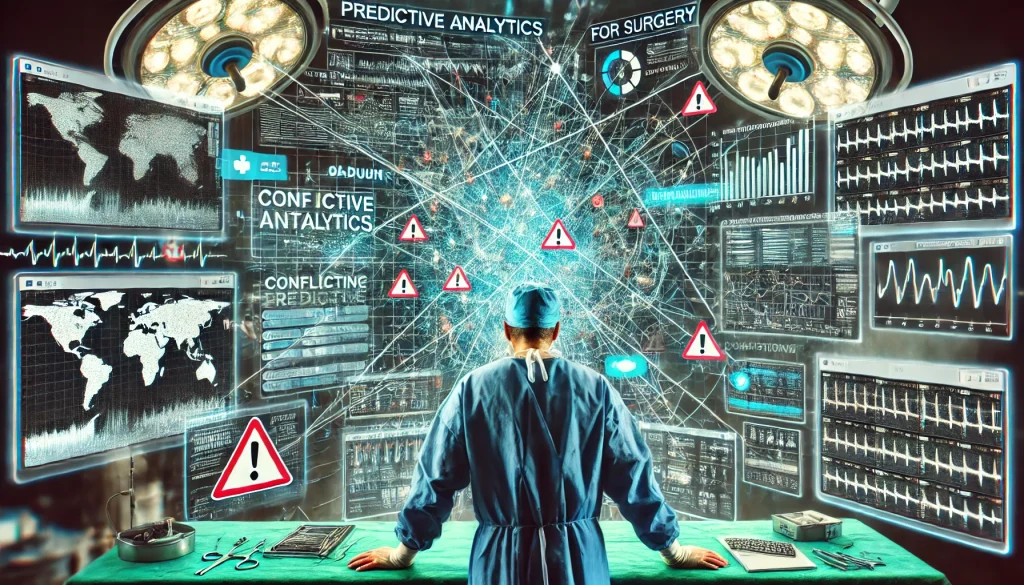
Biased Training Data
Predictive models are only as good as the data they are trained on. If the training data is biased—perhaps over-representing certain demographic groups or under-representing others—the resulting predictions may be skewed. This could lead to disparities in treatment outcomes, with certain patient groups receiving less accurate predictions. Regular auditing of predictive models is essential to identify and correct biases, ensuring fair treatment across all patient demographics.
Ethical Implications of Bias
Beyond technical concerns, algorithmic bias raises significant ethical questions. If a predictive model consistently favors one group over another, it could exacerbate existing healthcare disparities. Addressing these biases is not just a technical challenge but an ethical imperative that requires ongoing vigilance and adjustment.
Integration with Clinical Workflows
Complexity of Implementation
Integrating predictive analytics into existing clinical workflows can be a complex process. Surgeons and medical staff need to be trained on how to interpret and act on predictive insights without disrupting their established routines. Effective training programs and user-friendly interfaces are key to ensuring that predictive tools are seamlessly adopted into everyday practice.
Resistance to Change
Healthcare professionals may be resistant to adopting new technologies, particularly if they feel that predictive analytics tools could undermine their expertise or autonomy. Building trust in these tools requires demonstrating their value through clear, practical benefits in real-world settings.
Real-Time Data Processing
Challenges in Real-Time Analysis
One of the most promising aspects of predictive analytics in surgery is the potential for real-time decision-making support. However, processing vast amounts of data in real time poses significant technical challenges. The need for high-speed data processing and robust computational infrastructure is essential to deliver actionable insights during surgery without delays.
Balancing Speed and Accuracy
In the rush to provide real-time insights, there is a risk that the accuracy of predictions could be compromised. Striking the right balance between speed and accuracy is crucial, ensuring that predictions are not only timely but also reliable enough to inform critical surgical decisions.
Patient Privacy Concerns
Data Security Risks
The use of predictive analytics involves handling large amounts of sensitive patient data. Ensuring the security of this data is paramount to prevent breaches that could compromise patient privacy. Implementing strong encryption protocols and secure data storage solutions is necessary to protect patient information.
Informed Consent
Patients must be fully informed about how their data will be used in predictive models. This includes understanding the potential risks and benefits of predictive analytics. Obtaining informed consent is not just a legal requirement but also a crucial step in maintaining patient trust.
Regulatory and Legal Challenges
Navigating Complex Regulations
The use of predictive analytics in surgery must comply with a complex web of regulations, including those related to data protection, patient rights, and medical device approval. Healthcare providers need to stay informed about these regulations to avoid legal pitfalls and ensure that their use of predictive tools is fully compliant.
Liability Issues
Predictive analytics introduces new questions around liability. If a prediction leads to a negative outcome, determining who is responsible—the surgeon, the healthcare provider, or the developers of the predictive model—can be challenging. Clear guidelines on liability are needed to navigate these issues effectively.
Balancing Predictive Analytics with Clinical Judgment
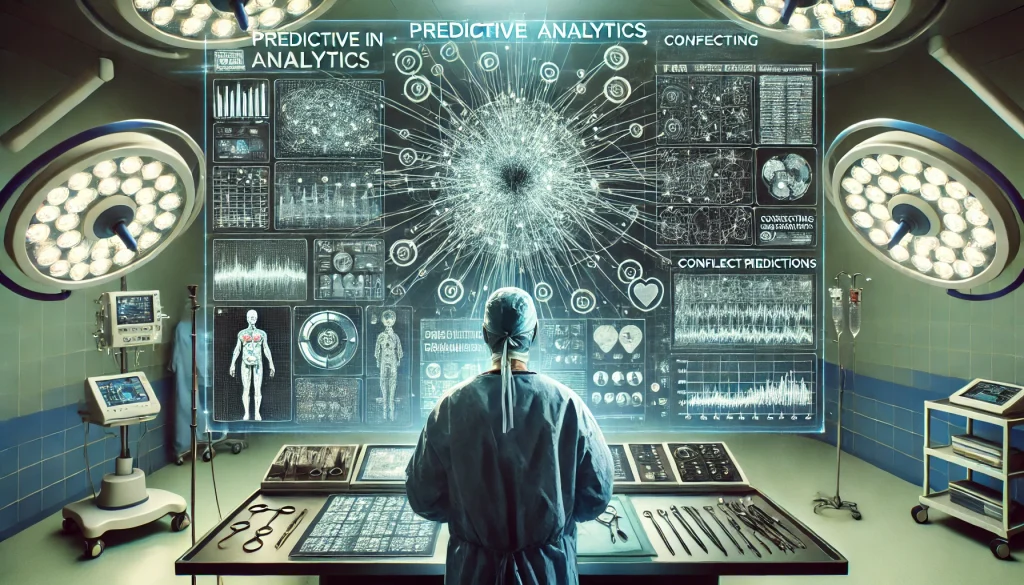
Over-Reliance on Predictive Tools
While predictive analytics provides valuable insights, there is a risk that healthcare providers might over-rely on these tools, potentially at the expense of clinical judgment. Maintaining a balanced approach where predictive analytics complements, rather than replaces, human expertise is essential for optimal patient care.
Ensuring Clinical Oversight
To prevent over-reliance, predictive analytics should be integrated as a decision-support tool rather than a decision-making tool. Surgeons must retain oversight of the predictive process, using their experience and intuition to guide final decisions.
Cost and Resource Allocation
High Implementation Costs
The implementation of predictive analytics requires significant investment in technology, training, and infrastructure. For many healthcare facilities, particularly those with limited resources, these costs can be prohibitive. Evaluating the return on investment is crucial to justify the expense and ensure that the benefits outweigh the costs.
Resource Allocation Challenges
Predictive analytics can help optimize the use of resources in surgical settings, but it also requires significant resources to function effectively. Balancing these demands is a challenge that requires careful planning and prioritization.
Future Ethical Considerations
Evolving Ethical Standards
As predictive analytics technology evolves, so too must the ethical standards that govern its use. New challenges will arise, such as those related to AI autonomy and data ownership, requiring ongoing ethical scrutiny and the development of new guidelines.
Global Disparities
Predictive analytics could exacerbate global healthcare disparities if access to these tools is limited to wealthier countries or institutions. Addressing these disparities requires a commitment to making predictive analytics accessible to a broader range of healthcare providers worldwide.
Conclusion
The challenges of integrating predictive analytics into surgery are significant but not insurmountable. By addressing issues related to data quality, bias, workflow integration, and ethical considerations, we can unlock the full potential of predictive analytics to improve surgical outcomes. The future of surgery is data-driven, but it must also be ethical, inclusive, and centered on patient care.
Top 10 Real-Life Use Cases: Challenges in Predictive Analytics for Surgery
1. Inconsistent Data Collection Across Healthcare Providers
Use Case:
Different healthcare providers often collect patient data using varied methods and standards. This inconsistency can result in incomplete or inaccurate data being fed into predictive models, leading to unreliable predictions.
Benefits:
- Improved Accuracy: Standardizing data collection processes across institutions ensures that the data used in predictive models is reliable, leading to more accurate surgical predictions.
- Better Collaboration: Consistent data collection facilitates collaboration between healthcare providers, enabling shared insights and improved patient care.
2. Algorithmic Bias in Predictive Models
Use Case:
Predictive models trained on biased data may generate skewed predictions that do not accurately reflect the needs of all patient groups. This can result in unequal treatment outcomes, particularly for underrepresented populations.
Benefits:
- Equitable Care: Identifying and correcting biases in predictive models ensures that all patient groups receive fair and accurate predictions, leading to more equitable surgical outcomes.
- Enhanced Model Performance: Reducing bias improves the overall performance of predictive models, making them more reliable for a broader range of patients.
3. Integration with Existing Clinical Workflows
Use Case:
Integrating predictive analytics into existing clinical workflows can be challenging, particularly if it disrupts established processes. Surgeons and medical staff may struggle to incorporate new predictive tools without affecting the efficiency of surgical procedures.
Benefits:
- Seamless Adoption: Proper integration of predictive analytics tools ensures that they complement, rather than disrupt, existing workflows, leading to smoother surgical processes.
- Increased Utilization: When predictive tools are easy to use and fit naturally into clinical routines, they are more likely to be adopted and utilized effectively by healthcare professionals.
4. Real-Time Data Processing Limitations
Use Case:
Real-time predictive analytics requires the rapid processing of vast amounts of data during surgery. However, the technological infrastructure needed to support this can be complex and expensive, and any delays in data processing can compromise the value of real-time insights.
Benefits:
- Timely Decision-Making: Effective real-time data processing provides surgeons with critical insights during surgery, enabling more informed decisions and better patient outcomes.
- Enhanced Surgical Precision: With real-time feedback, surgeons can adjust their techniques on-the-fly, improving the precision and safety of surgical procedures.
5. Patient Privacy and Data Security
Use Case:
The use of predictive analytics involves handling sensitive patient data, raising concerns about privacy and security. Protecting this data from breaches is essential to maintaining patient trust and complying with legal requirements.
Benefits:
- Trust and Confidence: Strong data security measures ensure that patients feel confident that their personal information is safe, fostering trust in the healthcare system.
- Legal Compliance: Adhering to data protection regulations prevents legal issues and protects healthcare providers from potential lawsuits related to data breaches.
6. Legal and Regulatory Compliance
Use Case:
Predictive analytics in surgery must comply with various legal and regulatory frameworks, which can be complex and vary by region. Navigating these regulations is challenging but essential to ensure that predictive tools are used ethically and legally.
Benefits:
- Risk Mitigation: Compliance with legal standards reduces the risk of legal action against healthcare providers and ensures that predictive analytics is used responsibly.
- Standardization: Adhering to regulations helps establish standardized practices in predictive analytics, improving overall care quality and patient safety.
7. Over-Reliance on Predictive Tools
Use Case:
There is a risk that surgeons might over-rely on predictive analytics, potentially sidelining their own clinical judgment. This could lead to decisions that are too heavily based on model predictions rather than a comprehensive assessment of the patient’s condition.
Benefits:
- Balanced Decision-Making: Ensuring that predictive analytics is used as a support tool, rather than a decision-making authority, maintains the critical role of human judgment in surgery.
- Improved Outcomes: A balanced approach that combines predictive insights with clinical expertise leads to better surgical outcomes for patients.
8. High Implementation Costs
Use Case:
Implementing predictive analytics requires significant investment in technology, infrastructure, and training. For many healthcare facilities, especially those with limited resources, these costs can be a barrier to adoption.
Benefits:
- Cost-Effective Solutions: By carefully evaluating the return on investment, healthcare providers can prioritize the adoption of predictive tools that offer the most significant benefits, ensuring that resources are used efficiently.
- Long-Term Savings: While the initial costs may be high, the long-term benefits of improved surgical outcomes and optimized resource use can lead to significant cost savings.
9. Managing Data Overload
Use Case:
Predictive analytics involves processing large volumes of data, which can be overwhelming for both the technology and the healthcare providers who need to interpret it. Managing this data effectively is a major challenge.
Benefits:
- Focused Insights: Implementing strategies to filter and prioritize data ensures that only the most relevant information is presented to surgeons, reducing cognitive overload.
- Efficient Use of Technology: Proper data management enhances the performance of predictive tools, ensuring that they operate efficiently and provide timely insights.
10. Future Ethical Considerations
Use Case:
As predictive analytics continues to evolve, new ethical challenges will arise, such as issues related to AI autonomy, data ownership, and global disparities in access to technology. Addressing these challenges proactively is essential.
Benefits:
- Proactive Ethics Management: By anticipating future challenges, healthcare providers can develop ethical guidelines and best practices that evolve alongside the technology.
- Sustained Trust: Addressing ethical concerns head-on ensures that predictive analytics continues to be a trusted tool in surgical practice, maintaining patient and provider confidence in its use.
FAQ on Challenges in Predictive Analytics for Surgery
What is the biggest challenge in using predictive analytics in surgery?
The biggest challenge is ensuring data quality. Inconsistent or incomplete data can lead to inaccurate predictions, which could compromise patient safety and surgical outcomes. Standardizing data collection practices across healthcare providers is crucial to address this issue.
How can bias in predictive models affect surgical outcomes?
Bias in predictive models can result in skewed predictions that may favor certain patient groups over others. This can lead to unequal treatment and potentially poorer outcomes for underrepresented populations. Regular audits and adjustments to these models are necessary to mitigate bias.
Why is integrating predictive analytics into clinical workflows difficult?
Integrating predictive analytics into existing clinical workflows can be challenging because it often requires changes to established practices. Training healthcare professionals on how to effectively use these tools without disrupting their routines is essential for successful integration.
How does real-time data processing impact predictive analytics in surgery?
Real-time data processing is critical for providing actionable insights during surgery, but it requires robust technological infrastructure. Any delays in processing can reduce the usefulness of the predictions, so balancing speed with accuracy is a significant challenge.
What are the privacy concerns with predictive analytics in surgery?
Predictive analytics involves handling sensitive patient data, raising concerns about privacy and data security. Ensuring that this data is protected from breaches is essential to maintaining patient trust and complying with legal requirements.
How do legal regulations affect the use of predictive analytics in surgery?
Legal regulations govern how predictive analytics can be used, particularly regarding data protection and patient rights. Healthcare providers must navigate these regulations carefully to avoid legal issues and ensure ethical use of predictive tools.
Can surgeons rely too much on predictive analytics?
Yes, there is a risk of over-reliance on predictive analytics, which could overshadow the surgeon’s clinical judgment. Predictive tools should be used to support, not replace, the expertise of healthcare professionals.
Why are implementation costs a challenge for predictive analytics in surgery?
The implementation of predictive analytics requires significant investment in technology, infrastructure, and training. These costs can be prohibitive, especially for smaller or under-resourced healthcare facilities, making it a challenge to adopt these tools widely.
How can data overload be managed in predictive analytics?
Managing data overload involves filtering and prioritizing information so that only the most relevant data is presented to surgeons. This helps reduce cognitive burden and ensures that the predictive tools are effective in supporting surgical decisions.
What ethical concerns arise with the future of predictive analytics?
As predictive analytics evolves, new ethical concerns will emerge, such as AI autonomy, data ownership, and disparities in access to technology. Addressing these challenges requires ongoing ethical scrutiny and the development of updated guidelines.
How can we ensure patient consent with predictive analytics?
Ensuring informed consent involves clearly communicating to patients how their data will be used in predictive analytics. Patients should understand the potential risks and benefits before agreeing to have their data included in predictive models.
What role does human oversight play in predictive analytics for surgery?
Human oversight remains crucial in predictive analytics. While predictive tools provide valuable insights, surgeons must continue to use their judgment and experience to make final decisions, ensuring that patient care remains personalized.
How can bias in predictive models be addressed?
Bias in predictive models can be addressed by regularly auditing the models and adjusting them to ensure they reflect a diverse patient population. This helps prevent skewed predictions and ensures that all patients receive fair and accurate care.
Why is real-time feedback important in predictive analytics for surgery?
Real-time feedback is important because it allows surgeons to adjust their techniques during surgery based on current data. This can lead to better outcomes, but it requires that predictive tools process data quickly and accurately.
What are the long-term challenges of predictive analytics in surgery?
Long-term challenges include keeping up with evolving technology, ensuring that predictive tools remain ethical and fair, and addressing disparities in access. Ongoing education and adaptation will be necessary to overcome these challenges and maximize the benefits of predictive analytics in surgery.