Future Trends in Predictive Analytics for Complication Prediction
- AI and Machine Learning: Greater integration for more precise predictions.
- Real-Time Monitoring: Enhanced real-time data analysis during treatment.
- Broader Application: Expanded use across various medical specialties.
- Ethical AI: Focus on developing fair and transparent predictive models.
- Improved Patient Outcomes: Continued advancements leading to better patient care and safety.
Introduction
Predictive analytics has become an essential tool in modern healthcare, particularly in predicting complications before they arise. By analyzing vast amounts of patient data, predictive models can identify risks and provide healthcare providers with crucial insights to take proactive measures. This article explores the role of predictive analytics in complication prediction, detailing its applications, benefits, and future implications for patient care.
The Role of Predictive Analytics in Complication Prediction
Predictive analytics plays a vital role in healthcare by anticipating complications that could arise during or after medical procedures. By leveraging historical data, real-time monitoring, and advanced algorithms, predictive analytics enables healthcare providers to mitigate risks and improve patient outcomes.
- Early Risk Identification: Predictive models analyze patient history, comorbidities, and current health status to identify potential complications early.
- Proactive Interventions: With insights from predictive analytics, healthcare providers can implement targeted interventions to prevent complications.
- Data-Driven Decisions: The use of data-driven insights enhances decision-making, ensuring that every patient receives care tailored to their specific risks.
Applications of Predictive Analytics in Complication Prediction
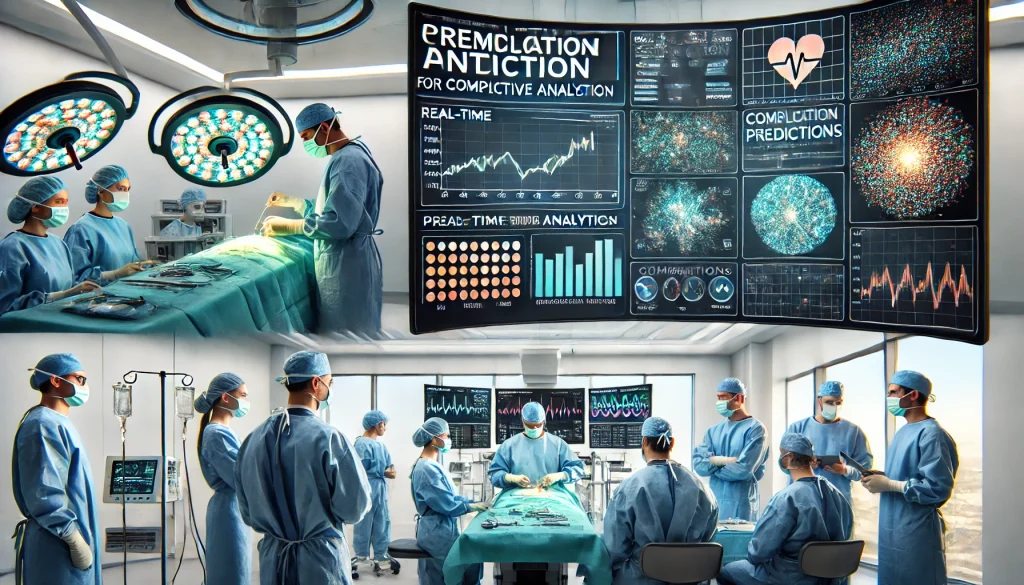
Predictive analytics is applied across various aspects of healthcare, particularly in predicting and preventing complications in different medical contexts. These applications are crucial in minimizing risks and improving patient outcomes.
Surgical Complication Prediction
In surgical settings, predictive analytics is used to forecast potential complications such as bleeding, infection, or adverse reactions to anesthesia. By assessing preoperative data and intraoperative monitoring, healthcare teams can anticipate issues and take preventive measures.
- Preoperative Risk Assessment: Predictive models evaluate factors such as patient age, medical history, and type of surgery to predict potential risks.
- Intraoperative Monitoring: Real-time data analysis during surgery helps in detecting early signs of complications, allowing for immediate intervention.
- Postoperative Care Planning: Based on predictive insights, postoperative care is tailored to mitigate risks identified before and during surgery.
Cardiovascular Complication Prediction
Cardiovascular patients are at high risk for complications such as heart attacks, strokes, and arrhythmias. Predictive analytics plays a crucial role in monitoring these patients and predicting potential complications.
- Monitoring Vital Signs: Predictive models continuously analyze vital signs to detect early indicators of cardiovascular events.
- Customized Treatment Plans: Patients receive personalized treatment plans based on their predicted risk of complications.
- Reduced Mortality Rates: By predicting and preventing cardiovascular complications, predictive analytics contributes to lower mortality rates among high-risk patients.
Infection Control and Prevention
Hospital-acquired infections (HAIs) are a significant concern in healthcare settings. Predictive analytics helps in identifying patients at risk of infections such as pneumonia, sepsis, or surgical site infections (SSIs).
- Risk Factor Analysis: Predictive models analyze factors like immune status, hospital stay duration, and previous infections to predict HAI risk.
- Targeted Preventive Measures: High-risk patients receive specialized care, including prophylactic antibiotics and enhanced hygiene protocols.
- Improved Infection Outcomes: With early intervention, the incidence of HAIs is reduced, leading to better patient outcomes and shorter hospital stays.
Benefits of Predictive Analytics for Complication Prediction
The integration of predictive analytics into healthcare for complication prediction offers numerous benefits. These advantages not only improve patient care but also contribute to more efficient healthcare delivery.
Enhanced Patient Safety
Predictive analytics enhances patient safety by providing early warnings about potential complications. This allows healthcare providers to act swiftly, preventing issues before they escalate.
- Early Detection: Potential complications are identified before they manifest, enabling timely intervention.
- Reduced Adverse Events: By predicting risks, healthcare teams can take preventive steps, leading to fewer adverse events.
- Improved Patient Confidence: Patients feel more secure knowing that their care includes proactive risk management.
Optimized Resource Allocation
Predictive analytics helps in optimizing the use of healthcare resources by identifying patients who need the most attention. This ensures that critical resources are allocated efficiently, improving overall care delivery.
- Prioritizing High-Risk Patients: Resources are focused on patients who are most likely to experience complications.
- Efficient Care Delivery: By anticipating complications, healthcare teams can prepare and allocate resources in advance, reducing waste and delays.
- Cost Savings: Preventing complications reduces the need for additional treatments, surgeries, or extended hospital stays, leading to significant cost savings.
Personalized Patient Care
Predictive analytics allows for the customization of care plans based on individual patient risks. This personalized approach ensures that each patient receives the care most appropriate for their condition.
- Tailored Interventions: Care plans are designed to address specific risks identified through predictive analytics.
- Better Outcomes: Patients experience better outcomes when care is personalized to their unique health profiles.
- Enhanced Patient Experience: Personalized care leads to higher patient satisfaction and better overall healthcare experiences.
Challenges in Implementing Predictive Analytics for Complication Prediction
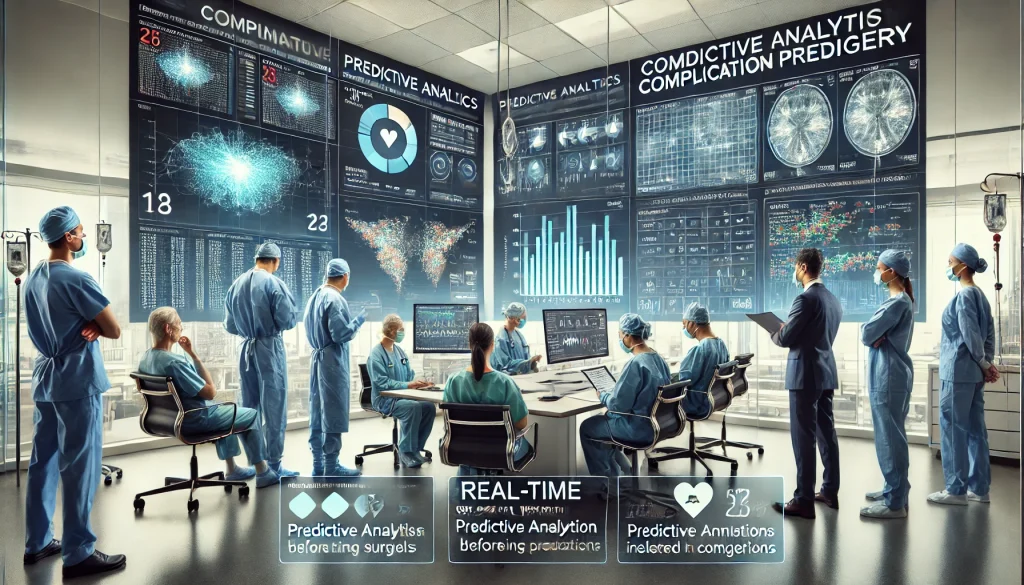
While the benefits are clear, implementing predictive analytics for complication prediction presents several challenges. Addressing these challenges is essential for maximizing the effectiveness of this technology in healthcare.
Data Integration and Quality
The accuracy of predictive models depends heavily on the quality and integration of data. Ensuring that data from various sources is accurate, consistent, and integrated into a single system is crucial for reliable predictions.
- Ensuring Data Accuracy: Accurate data is critical for reliable predictions, requiring robust data management practices.
- Integration of Multiple Data Sources: Healthcare providers must integrate data from electronic health records (EHRs), wearable devices, and other monitoring systems.
- Handling Data Gaps: Incomplete or missing data can lead to inaccurate predictions, so strategies must be in place to manage these gaps.
Ethical and Privacy Concerns
The use of predictive analytics raises ethical and privacy concerns, particularly regarding patient data security and the potential for bias in predictive models.
- Protecting Patient Privacy: Ensuring that patient data is secure and used in compliance with privacy regulations is paramount.
- Addressing Bias: Predictive models must be carefully designed and tested to avoid bias, ensuring that all patients receive fair and equitable care.
- Informed Consent: Patients should be informed about how their data will be used in predictive analytics and have the opportunity to consent.
Training and Adoption
For predictive analytics to be effective, healthcare providers need to be trained in its use. Adoption can be challenging if teams are not familiar with the technology or if it disrupts established workflows.
- Training Healthcare Providers: Providers need comprehensive training to use predictive analytics tools effectively and interpret the data generated.
- Encouraging Adoption: Demonstrating the benefits of predictive analytics can help overcome resistance and encourage widespread use.
- Continuous Support: Ongoing support and updates are necessary to keep healthcare teams proficient in using predictive analytics.
Future of Predictive Analytics for Complication Prediction
The future of predictive analytics for complication prediction is promising, with ongoing advancements in technology and data science poised to enhance its impact on healthcare.
- AI and Machine Learning Integration: The integration of AI and machine learning will enhance the accuracy and capabilities of predictive models.
- Real-Time Data Analysis: Future developments will enable even more precise real-time monitoring and prediction of complications.
- Wider Application: Predictive analytics will be applied more broadly across different medical specialties, benefiting a wider range of patients.
- Ethical AI Development: Continued focus on developing ethical AI will ensure that predictive models are fair, transparent, and beneficial for all patients.
Conclusion
Predictive analytics is transforming the way healthcare providers approach complication prediction, offering a powerful tool for improving patient safety, optimizing resources, and delivering personalized care. By anticipating potential complications before they arise, healthcare teams can take proactive measures to prevent adverse outcomes, leading to better patient care and more efficient healthcare delivery. As technology continues to advance, the role of predictive analytics in complication prediction will only grow, offering new opportunities to enhance the quality and effectiveness of medical treatment.
Top 10 Real-Life Use Cases: Predictive Analytics for Complication Prediction
1. Reducing Surgical Site Infections
Case Study: Predicting Infection Risks in Post-Surgery Patients
Predictive analytics is used to identify patients at risk for surgical site infections (SSIs) by analyzing factors such as patient history, surgical techniques, and wound care practices.
Benefits:
- Targeted Prevention: High-risk patients receive tailored preventive measures, including antibiotics and enhanced wound care.
- Faster Recovery: By preventing infections, patients experience quicker recoveries with fewer complications.
- Lower Healthcare Costs: Reducing the incidence of SSIs leads to decreased hospital readmissions and lower overall healthcare costs.
2. Preventing Cardiovascular Events
Case Study: Monitoring Postoperative Heart Complications
In patients recovering from surgery, predictive analytics monitors vital signs and other indicators to predict the likelihood of heart-related complications such as arrhythmias or heart attacks.
Benefits:
- Early Detection: Potential heart issues are identified early, allowing for immediate intervention.
- Customized Treatment: Patients receive personalized care plans to address specific cardiovascular risks.
- Reduced Mortality Rates: Early intervention and monitoring significantly decrease the risk of life-threatening heart events.
3. Avoiding Respiratory Complications
Case Study: Identifying High-Risk Patients for Pneumonia
Predictive analytics helps identify patients at risk for postoperative pneumonia, particularly those with underlying respiratory conditions or those who have undergone major surgery.
Benefits:
- Proactive Respiratory Care: At-risk patients receive early interventions such as respiratory therapy or prophylactic antibiotics.
- Decreased ICU Admissions: Early management reduces the need for intensive care and mechanical ventilation.
- Improved Patient Outcomes: Patients experience fewer respiratory complications, leading to better overall recovery.
4. Managing Blood Clot Risks
Case Study: Predicting Thrombosis in Post-Surgical Patients
Predictive models assess the likelihood of patients developing deep vein thrombosis (DVT) or pulmonary embolism (PE) after surgery.
Benefits:
- Preventive Measures: High-risk patients receive anticoagulants or mechanical compression devices to prevent clots.
- Lowered Complication Rates: Early intervention reduces the incidence of DVT and PE, which are leading causes of morbidity after surgery.
- Increased Patient Safety: Patients are monitored closely, ensuring timely response to any signs of clot formation.
5. Minimizing Adverse Drug Reactions
Case Study: Predicting Patient Responses to Medications
Predictive analytics is used to forecast how patients will respond to specific medications, particularly in the postoperative period when drug reactions can complicate recovery.
Benefits:
- Personalized Medication Plans: Patients receive drugs that are most likely to be effective and least likely to cause adverse reactions.
- Reduced Side Effects: By predicting and avoiding drugs with high risk of side effects, patient comfort and safety are improved.
- Better Recovery: Patients experience smoother recoveries with fewer interruptions due to drug-related complications.
6. Preventing Postoperative Delirium
Case Study: Identifying Elderly Patients at Risk
Elderly patients are at a higher risk of developing postoperative delirium, which can complicate recovery. Predictive analytics identifies those most likely to be affected.
Benefits:
- Preventive Strategies: High-risk patients are provided with cognitive support, environmental modifications, and medication adjustments.
- Shortened Hospital Stays: By preventing delirium, patients recover more quickly and are discharged sooner.
- Enhanced Quality of Life: Elderly patients maintain better cognitive function and overall health post-surgery.
7. Managing Diabetes in Postoperative Care
Case Study: Controlling Blood Glucose Levels
For diabetic patients, predictive analytics helps manage blood glucose levels during the postoperative period, reducing the risk of complications such as infections and poor wound healing.
Benefits:
- Optimized Insulin Therapy: Patients receive customized insulin regimens based on their predicted glucose levels.
- Reduced Complication Rates: Proper glucose management leads to fewer infections and better wound healing.
- Improved Long-Term Outcomes: Patients experience better long-term health by maintaining stable blood sugar levels during recovery.
8. Predicting Renal Failure
Case Study: Monitoring Kidney Function After Major Surgery
Predictive analytics monitors patients for signs of acute kidney injury (AKI) following major surgery, particularly in those with pre-existing kidney conditions.
Benefits:
- Early Intervention: Potential kidney issues are identified early, allowing for timely treatment and fluid management.
- Preventing Long-Term Damage: Early management reduces the risk of long-term kidney damage or chronic kidney disease.
- Enhanced Recovery: Patients experience fewer complications related to kidney function, leading to faster recovery times.
9. Avoiding Gastrointestinal Complications
Case Study: Predicting Postoperative Ileus
Predictive models are used to identify patients at risk for postoperative ileus, a condition where the intestines do not function properly after surgery.
Benefits:
- Targeted Interventions: Patients at risk receive early interventions such as medication or dietary modifications to stimulate bowel function.
- Reduced Hospital Stays: Preventing ileus leads to shorter hospital stays and quicker returns to normal activity.
- Better Patient Outcomes: Patients experience less discomfort and quicker recoveries when ileus is effectively managed.
10. Reducing Postoperative Nausea and Vomiting (PONV)
Case Study: Identifying Patients Prone to PONV
Predictive analytics identifies patients at risk for postoperative nausea and vomiting, particularly those with a history of motion sickness or previous PONV.
Benefits:
- Personalized Antiemetic Therapy: Patients receive tailored antiemetic regimens based on their risk profile.
- Improved Recovery Experience: Effective management of PONV leads to a more comfortable recovery with less disruption.
- Decreased Need for Readmission: By controlling PONV, patients are less likely to require readmission for complications related to dehydration or aspiration.
FAQ About Predictive Analytics for Complication Prediction
How does predictive analytics help in predicting complications?
Predictive analytics uses patient data, such as medical history and current health metrics, to forecast potential complications. By identifying risks early, healthcare providers can take preventive actions to avoid adverse outcomes.
Can predictive analytics prevent surgical complications?
Yes, predictive analytics can identify patients at higher risk for complications during or after surgery. This allows healthcare teams to implement specific measures, such as adjusting surgical techniques or providing additional monitoring.
What types of complications can predictive analytics predict?
Predictive analytics can forecast a wide range of complications, including infections, cardiovascular events, respiratory issues, blood clots, and adverse drug reactions. It helps in assessing risks based on individual patient profiles.
Is predictive analytics used only in high-risk surgeries?
Predictive analytics is valuable in both high-risk and routine surgeries. It provides insights that help improve outcomes for all patients by tailoring care plans to individual needs and risks.
How reliable are predictions made by predictive analytics?
The reliability of predictions depends on the quality of data and the models used. While not perfect, predictive analytics provides valuable insights that guide clinical decisions and improve patient safety.
Can predictive analytics help with postoperative care?
Yes, predictive analytics plays a crucial role in postoperative care by identifying patients at risk for complications after surgery. It guides care teams in providing targeted interventions during recovery.
What data is required for predictive analytics to work effectively?
Predictive analytics requires accurate and comprehensive patient data, including medical history, lab results, vital signs, and any relevant real-time monitoring data. The more complete the data, the better the predictions.
Is patient privacy protected when using predictive analytics?
Patient privacy is a top priority when using predictive analytics. All data used must be handled securely and in compliance with privacy regulations to protect patient information.
How does predictive analytics benefit patients?
Patients benefit from predictive analytics through more personalized care, early intervention to prevent complications, and overall improved outcomes. It allows for proactive management of potential health risks.
Can predictive analytics reduce hospital readmissions?
Predictive analytics can identify patients at risk for complications that could lead to readmission. By addressing these risks early, healthcare providers can reduce the likelihood of patients needing to return to the hospital.
What challenges are associated with implementing predictive analytics?
Challenges include ensuring data accuracy, integrating predictive tools with existing healthcare systems, and training healthcare providers to use the technology effectively. Overcoming these challenges is key to realizing the full benefits.
How is predictive analytics integrated into the healthcare workflow?
Predictive analytics is integrated through software systems that analyze patient data and provide actionable insights to healthcare providers. These insights are used to inform clinical decisions and improve patient care.
Can predictive analytics be used in emergency situations?
Yes, predictive analytics can be used in emergency situations to assess risks and guide immediate care decisions. It helps healthcare teams prioritize interventions and manage patient care under pressure.
Is predictive analytics applicable to all medical specialties?
Predictive analytics can be applied across various medical specialties, from surgery and cardiology to oncology and obstetrics. Its versatility makes it a valuable tool in many areas of healthcare.
What is the future of predictive analytics in healthcare?
The future of predictive analytics in healthcare includes more advanced AI-driven models, real-time monitoring, and broader adoption across different medical fields. These advancements will continue to improve patient outcomes and care delivery.