Advancing Predictive Models in Surgical Outcomes
- AI Integration: Enhanced accuracy through AI collaboration.
- Real-Time Predictions: Dynamic adjustments during surgery.
- Global Standardization: Universal application and consistency.
- Personalized Surgery: Tailored procedures for individual patients.
- Continuous Learning: Models that improve with ongoing data input.
Introduction
In modern healthcare, predictive models are revolutionizing how we approach surgical outcomes. These models, powered by machine learning and vast datasets, enable healthcare professionals to anticipate risks, plan interventions, and ultimately improve patient care. This article delves into the intricate world of predictive models, offering a comprehensive understanding of their application, benefits, and challenges in surgery.
The Role of Predictive Models in Surgery
Understanding Predictive Models
Predictive models use algorithms to analyze historical data and identify patterns. These models can forecast the likelihood of various surgical outcomes, such as complications, recovery times, and mortality rates.
How Predictive Models Are Applied in Surgery
- Risk Stratification: Classifying patients into different risk categories based on their likelihood of experiencing complications.
- Decision Support: Assisting surgeons in choosing the most appropriate surgical technique or intervention.
- Personalized Medicine: Tailoring surgical procedures to the specific needs and risk profile of each patient.
Benefits of Predictive Models in Surgery
- Improved Patient Outcomes: Anticipating and mitigating risks before they occur.
- Optimized Resource Allocation: Allocating medical resources more efficiently based on predicted needs.
- Enhanced Surgical Planning: Providing surgeons with detailed insights into potential challenges during surgery.
Types of Predictive Models in Surgery
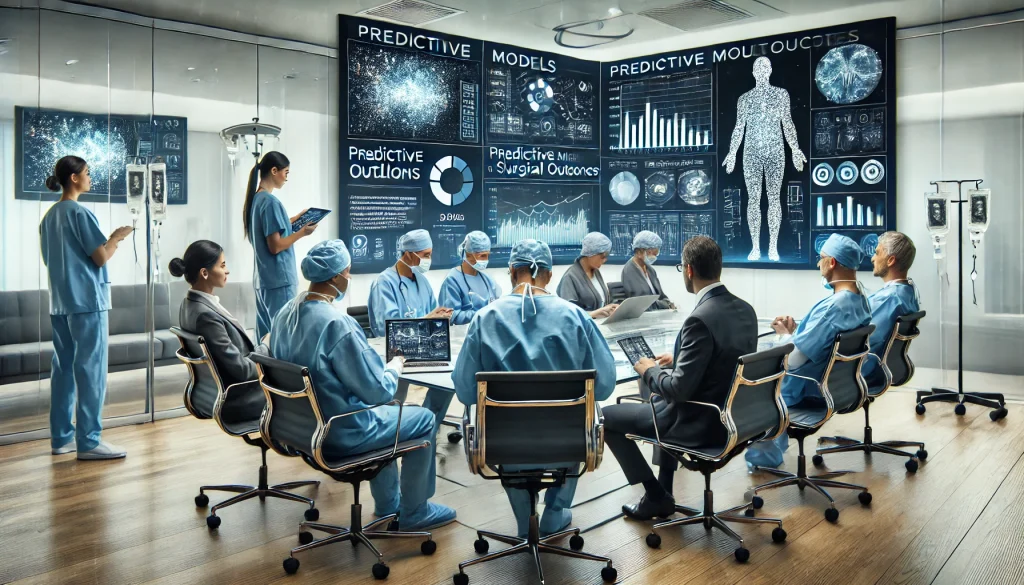
Logistic Regression Models
Logistic regression models are widely used for predicting binary outcomes, such as the likelihood of postoperative complications. They are relatively simple to implement and interpret.
Machine Learning Models
- Support Vector Machines (SVM): Effective in classifying complex datasets and predicting multiple outcomes.
- Random Forest: Combines multiple decision trees to improve accuracy and reduce overfitting.
- Neural Networks: Mimics the human brain to recognize patterns in large datasets, particularly useful in predicting complex surgical outcomes.
Survival Analysis Models
- Cox Proportional Hazards Model: Used to predict the time until an event, such as recurrence of disease post-surgery.
- Kaplan-Meier Estimator: Provides a visual representation of survival probabilities over time.
Bayesian Models
These models incorporate prior knowledge into the prediction process, allowing for more refined predictions, especially in cases with limited data.
Data Sources for Predictive Models in Surgery
Electronic Health Records (EHRs)
EHRs provide a wealth of patient information, including medical history, lab results, and previous surgical outcomes. This data is crucial for training predictive models.
Medical Imaging
- Radiology Reports: Used to predict outcomes based on preoperative imaging studies.
- Pathology Data: Helps in predicting the aggressiveness of tumors and the likelihood of recurrence.
Genomic Data
Genomic data is increasingly being integrated into predictive models to anticipate patient-specific responses to surgery and postoperative treatments.
Wearable Devices
Data from wearable devices can offer real-time insights into patient health, allowing for continuous monitoring and prediction of postoperative recovery.
Challenges in Implementing Predictive Models
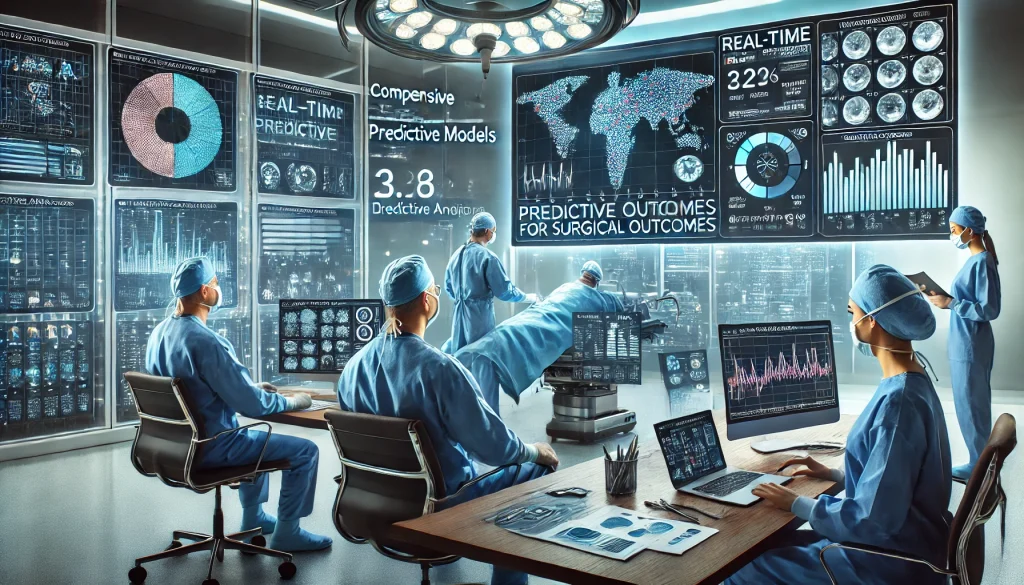
Data Quality and Availability
- Incomplete Data: Missing information can lead to inaccurate predictions.
- Data Silos: Fragmented data across different systems can hinder model accuracy.
Ethical Considerations
- Bias in Algorithms: Predictive models may reflect and amplify existing biases in healthcare data.
- Patient Privacy: Ensuring that predictive modeling respects patient confidentiality.
Integration with Clinical Workflow
Incorporating predictive models into everyday clinical practice requires significant changes in workflow and decision-making processes.
Case Studies: Successful Applications of Predictive Models in Surgery
Predicting Complications in Cardiovascular Surgery
Predictive models have been used to identify high-risk patients undergoing cardiovascular surgery, allowing for preemptive measures to be taken.
Enhanced Recovery After Surgery (ERAS) Protocols
Predictive models have informed ERAS protocols, leading to reduced recovery times and lower complication rates.
Precision Surgery in Oncology
In cancer surgery, predictive models have been used to tailor surgical approaches based on the predicted aggressiveness of the tumor.
The Future of Predictive Models in Surgery
Integration with Artificial Intelligence (AI)
The integration of AI with predictive models promises to enhance their accuracy and application in surgery, leading to more personalized and effective surgical care.
Real-Time Predictive Analytics
The future may see the development of real-time predictive models that can adjust predictions as new data becomes available during surgery.
Global Adoption and Standardization
Efforts are underway to standardize predictive models across healthcare systems globally, ensuring that all patients benefit from these advancements.
Conclusion
Predictive models are transforming surgical care, offering unprecedented insights into patient outcomes. As these models continue to evolve, they hold the promise of making surgery safer, more efficient, and more personalized. By embracing these technologies, healthcare providers can ensure that patients receive the best possible care, tailored to their unique needs and circumstances.
Top 10 Real-Life Use Cases of Predictive Models for Surgical Outcomes
1. Predicting Postoperative Complications in Cardiac Surgery
Benefits
Predictive models are extensively used in cardiac surgery to forecast potential postoperative complications such as heart failure, arrhythmias, or infections. By analyzing patient data like age, medical history, and preoperative tests, these models can identify high-risk patients. Surgeons can then tailor interventions, monitor patients closely, and allocate resources effectively, reducing the incidence of complications and improving overall survival rates.
2. Reducing Readmission Rates After Joint Replacement Surgery
Benefits
In joint replacement surgeries, particularly hip and knee replacements, predictive models are utilized to assess the likelihood of readmission due to complications like infections, blood clots, or prosthesis issues. By identifying patients at higher risk, healthcare teams can implement enhanced postoperative care plans, including physical therapy and closer monitoring. This proactive approach minimizes readmissions, optimizes resource use, and improves patient satisfaction.
3. Optimizing Resource Allocation in Emergency Surgeries
Benefits
In emergency surgical scenarios, where time is of the essence, predictive models help in determining the resources required, such as operating room availability, specialist requirements, and necessary equipment. These models analyze the urgency, type of surgery, and patient condition to forecast the demands. This ensures that critical resources are available when needed, reducing delays, improving patient outcomes, and enhancing the efficiency of emergency departments.
4. Tailoring Surgical Approaches in Cancer Treatment
Benefits
Predictive models are invaluable in oncology surgeries, particularly in determining the extent of tumor removal and the likelihood of recurrence. By analyzing tumor genetics, patient history, and previous treatment responses, these models guide surgeons in deciding the most effective surgical approach. This leads to more precise surgeries, better preservation of healthy tissue, and improved long-term survival rates for cancer patients.
5. Enhancing Recovery Protocols After Major Surgeries
Benefits
Enhanced Recovery After Surgery (ERAS) protocols are designed to speed up recovery and reduce complications. Predictive models contribute by forecasting individual patient recovery times and potential issues. For instance, in abdominal surgeries, these models predict which patients might benefit from more aggressive pain management or early mobilization. The result is a more personalized recovery plan, shorter hospital stays, and lower overall healthcare costs.
6. Forecasting Blood Loss in Trauma Surgeries
Benefits
In trauma surgeries, managing blood loss is critical. Predictive models assess factors like injury type, patient physiology, and historical data to estimate blood loss during surgery. This allows surgical teams to prepare adequate blood supplies, plan transfusions, and stabilize patients more effectively. Reducing the risk of hemorrhagic shock, these models contribute to better survival outcomes in trauma cases.
7. Personalizing Anesthesia Management
Benefits
Anesthesia is a critical aspect of any surgery, and predictive models are used to personalize anesthesia plans based on patient characteristics such as age, weight, and comorbidities. For example, in elderly patients or those with respiratory issues, predictive models can suggest optimal anesthesia types and dosages to minimize risks of postoperative delirium or respiratory complications. This leads to safer anesthesia management and quicker recovery from anesthesia-related effects.
8. Anticipating Surgical Site Infections (SSI)
Benefits
Surgical site infections (SSI) are a common postoperative complication. Predictive models evaluate risk factors such as the patient’s immune status, type of surgery, and hospital environment to predict the likelihood of an SSI. This allows for preemptive measures such as prophylactic antibiotics, enhanced sterilization procedures, and closer postoperative monitoring, ultimately reducing the incidence of infections and improving patient outcomes.
9. Predicting Outcomes of Bariatric Surgery
Benefits
In bariatric surgery, predictive models are employed to anticipate weight loss outcomes, potential nutritional deficiencies, and the likelihood of complications like dumping syndrome or gallstones. These models analyze patient demographics, lifestyle factors, and preoperative health conditions to customize surgical plans and postoperative care. This personalized approach helps achieve better long-term weight management and overall health improvements for patients undergoing bariatric procedures.
10. Improving Decision-Making in Organ Transplant Surgeries
Benefits
Organ transplant surgeries are complex, with numerous factors influencing outcomes, such as organ compatibility, patient health, and immune response. Predictive models help in assessing the suitability of organ donors, predicting graft survival rates, and anticipating rejection risks. By providing these insights, the models support more informed decision-making, improving the success rates of transplants and enhancing patient survival and quality of life post-transplant.
FAQ About Predictive Models for Surgical Outcomes
What are predictive models in surgical outcomes?
Predictive models in surgical outcomes are tools that analyze patient data to forecast potential results of surgery. These models use algorithms to predict complications, recovery times, and other important factors, helping healthcare providers make informed decisions.
How do predictive models help in reducing surgical risks?
These models identify patients who are at higher risk of complications by analyzing factors like medical history, age, and type of surgery. This allows healthcare teams to take preventative measures, such as closer monitoring or tailored interventions, to minimize risks.
Can predictive models be used in emergency surgeries?
Yes, predictive models are particularly useful in emergency surgeries. They quickly assess patient data to predict necessary resources, such as surgical equipment and specialist availability, ensuring that the patient receives timely and appropriate care.
Are predictive models only for complex surgeries?
No, predictive models are used across various types of surgeries, from routine procedures to more complex operations. They provide valuable insights that can improve patient outcomes, regardless of the surgery’s complexity.
How accurate are predictive models in surgery?
The accuracy of predictive models depends on the quality and quantity of data they are trained on. While no model can be 100% accurate, many have proven to be highly reliable in predicting outcomes, leading to better surgical planning and patient care.
What role do predictive models play in postoperative care?
Predictive models help tailor postoperative care by forecasting potential complications and recovery times. This allows healthcare providers to create personalized care plans, which can lead to faster recovery and reduced hospital stays.
How do predictive models improve surgical planning?
By analyzing patient data and predicting possible outcomes, these models provide surgeons with critical information that can influence the choice of surgical techniques, the timing of the surgery, and the allocation of resources, leading to better planning and execution.
Can predictive models be used for cancer surgeries?
Yes, predictive models are widely used in cancer surgeries to determine the best approach for tumor removal and to predict the likelihood of recurrence. This helps in making more precise surgical decisions and improves long-term outcomes for patients.
What types of data are used in predictive models for surgery?
Predictive models use a variety of data, including electronic health records, medical imaging, genomic data, and information from wearable devices. This data is analyzed to predict surgical outcomes and guide clinical decisions.
Are predictive models useful for anesthesiology?
Predictive models are valuable in anesthesiology as they help customize anesthesia plans based on patient-specific factors. This can reduce the risk of complications such as postoperative delirium or respiratory issues, particularly in vulnerable populations.
How do predictive models address the issue of surgical site infections?
These models predict the likelihood of surgical site infections by analyzing risk factors like patient immunity, the type of surgery, and the surgical environment. With these predictions, healthcare teams can implement preventive measures to reduce the incidence of infections.
Can predictive models be integrated into daily surgical practice?
Yes, predictive models are increasingly being integrated into daily practice. They provide real-time insights that help surgeons and medical teams make more informed decisions before, during, and after surgery, improving overall patient care.
Do predictive models take into account patient preferences?
While predictive models primarily focus on clinical data, they can be used alongside patient preferences to tailor surgical approaches and postoperative care, ensuring that the patient’s values and wishes are considered in the decision-making process.
What are the challenges in using predictive models for surgery?
Challenges include ensuring data quality, managing biases in the models, and integrating these tools into clinical workflows. Addressing these issues is crucial for the effective use of predictive models in surgical settings.
What is the future of predictive models in surgery?
The future likely involves greater integration with artificial intelligence, real-time analytics during surgery, and broader adoption across different healthcare systems. This will lead to even more personalized and precise surgical care.